Spotting the Signs of Disease Before You Even Know You’re Sick
It sounds like a sci-fi movie plot: What if smart computers could spot disease hours, or even years, before symptoms are clinically detectable? Yet, this story is far from fiction. Researchers at the University of Tennessee Health Science Center (UTHSC) are turning the improbable into possible, using artificial intelligence and machine learning to identify warning signs of disease long before they become visible to clinicians.
Novel technologies and applications incorporating artificial intelligence (AI) and machine learning (ML) are on the rise across all research fields. Just this year, the U.S. Patent Office issued updated guidelines clarifying patent eligibility criteria for AI/ML inventions. The new guidelines make UTRF better equipped than ever to protect AI and machine learning innovations and secure commercialization. These advancements provide critical value to the public – a fact clearly seen in Assistant Professor Rishi Kamaleswaran’s lab at UTHSC’s Center for Biomedical Informatics.
Rishi and his UTHSC colleagues are building algorithms with AI and machine learning to spot physiomarkers that predict the onset of disease. Essentially, the researchers use AI to identify neurologic dysregulation of the autonomic nervous system – the brain’s regulation of largely unconscious body functions like heart rate and respiratory rate of the lungs. Indicators of dysfunction in this neurological system may be invisible to the human eye, but can become apparent by capturing high-frequency data from EKGs and respiratory sensors and analyzing it for differential changes. Machine learning – a method of AI that teaches a computer through pattern recognition rather than explicit instruction – enables Rishi and his team to build new algorithms around the biomarker data that can detect these signals predicting the on-set of deadly diseases, such as sepsis.
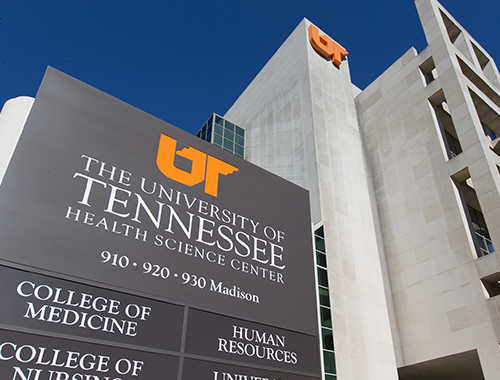
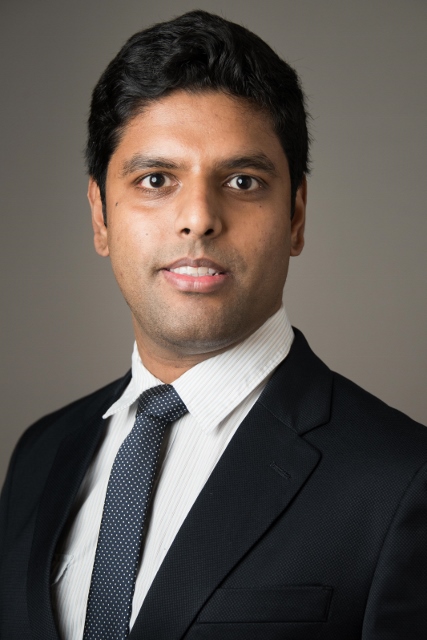
Sepsis is a dangerous condition in which the immune system reacts to an infection by attacking the body’s own tissue. The specific triggers of sepsis are still difficult to identify, and recovery depends on early detection and treatment, before vital organs begin to fail. Before building the new prediction model, Rishi studied the vital signs and other biomarkers of children admitted to the critical care unit of Memphis’ Le Bonheur Children’s Hospital, focusing on data from the 24 hours before alert systems flagged any of the children with sepsis. Applying machine learning, the researchers built a model from pattern recognition in this data that can predict signs of decompensation, one of the first indicators of sepsis. Though still in the trial phase, the outcomes are stunning: Rishi’s model can predict the onset of severe sepsis in children up to 8 hours earlier than current sepsis screening tools. After applying their AI approach to critically ill adults, the team built a model capable of predicting sepsis up to 17 hours earlier than conventional clinicians.
Rishi’s novel application of AI has the potential to give life-saving hours back to severe sepsis patients. But what about progressive neurological disorders, like Parkinson’s? Parkinson’s Disease involves the deterioration of nerve cells in the brain, which causes progressive neurological dysfunction, affecting the brain’s regulation of body systems and movement. Its cause is still unknown, as is a cure.
Applying AI and machine learning to EKG data, Rishi and his UTHSC colleagues found they could spot markers indicating Parkinson’s Disease before patients experienced any symptoms. In fact, their model found indicators of the disease up to two years before clinicians could diagnose it. The breakthrough is so promising that the Michael J. Fox Foundation recently bestowed a $500,000 grant on the researchers to demonstrate the new algorithm in a much larger study with current patients.
UTRF Vice President Richard Magid highlights that while still in its trial phase, Rishi’s research has the potential to help millions of patients and revolutionize diagnostic health care. “Across the spectrum of advancements in AI and machine learning, UTRF is here to support UT researchers like Rishi and is proud to play a role in bringing the possibilities of AI and machine learning out of the lab and into peoples’ daily lives,” he remarks.