SA-GAN Learning Package for Resilient Defense of Microgrids in Extreme Events
The Problem
When sequential extreme events (SEE) such as hurricanes and tornadoes arrive, severe damage to the power grid and loss of critical loads can occur. Conventional prediction models use inflexible stochastic programming or robust and data heavy optimization to handle these uncertain conditions. Newer AI models rely on limited and rare SEE event data, restricting their viability.
The Solution
Researchers at the University of Tennessee have developed the Self-Attention Generative Adversarial Network with a Deep Reinforced Learning package (SA-GAN-DRL), a novel AI supported decision assistance package, that can mitigate the impacts of extreme weather events by ensuring the survival of critical loads in a networked microgrid. This technology is a purely learning-based tool, which is adaptive to the changeable environment and can achieve model-free application. Specifically, it has a strong ability to learn from sequential data in a Markov decision process and provide a general basis to train the agent to learn proper network adjustment actions during changing system conditions.
Benefits
Benefit |
---|
Naturally adaptive and model-free for changing circumstances |
Improved grid resilience for networked micro-grids |
Efficient learning with limited data |
Allows operators to limit critical infrastructure downtime |
More Information
- Gregory Sechrist, JD
- Technology Manager, Multi Campus Office
- 865-974-1882 | gsechris@tennessee.edu
- UTRF Reference ID: 23077
- Patent Status:
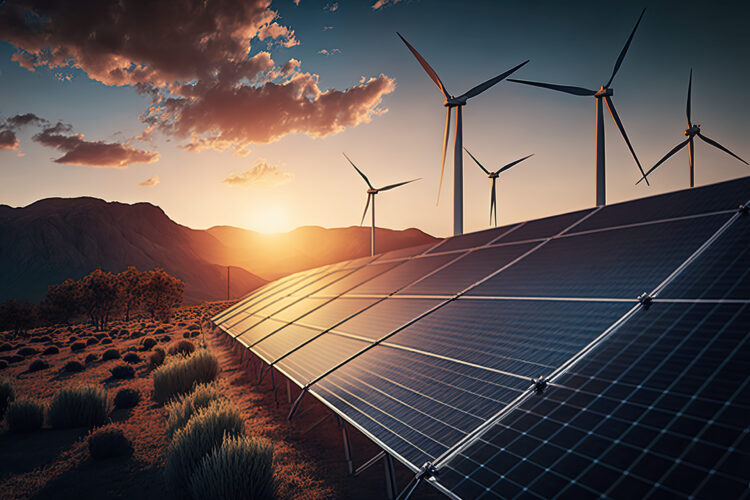
Innovators
Dr. Fangxing (Fran) Li
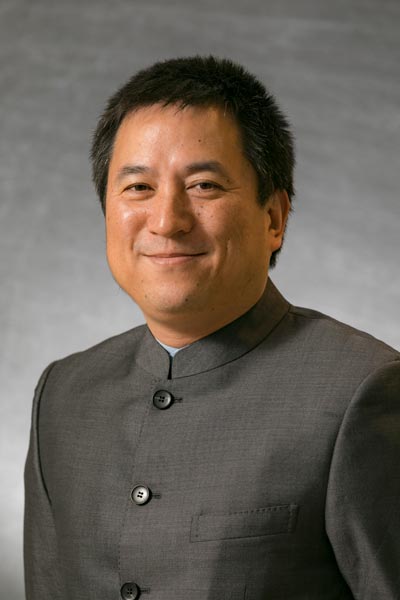
James W. McConnell Professor in the Department of Electrical Engineering and Computer Science (EECS) at The University of Tennessee Knoxville. He is also an adjunct researcher at the Oak Ridge National Laboratory (ORNL).
Dr. Fangxing (Fran) Li received his PhD from Virginia Tech University in 2001. His research interests include renewable energy integration, distributed energy resources, and power system computational methods. He is an adjunct researcher at Oak Ridge National Laboratory and the campus director of Center for Ultra-Wide-Area Resilient Electric Energy Transmission Networks (CURENT).
Read more about Dr. Fangxing (Fran) Li